Mastering Lead Qualification Strategies: How to Boost B2B Sales Efficiency and Conversion Rates
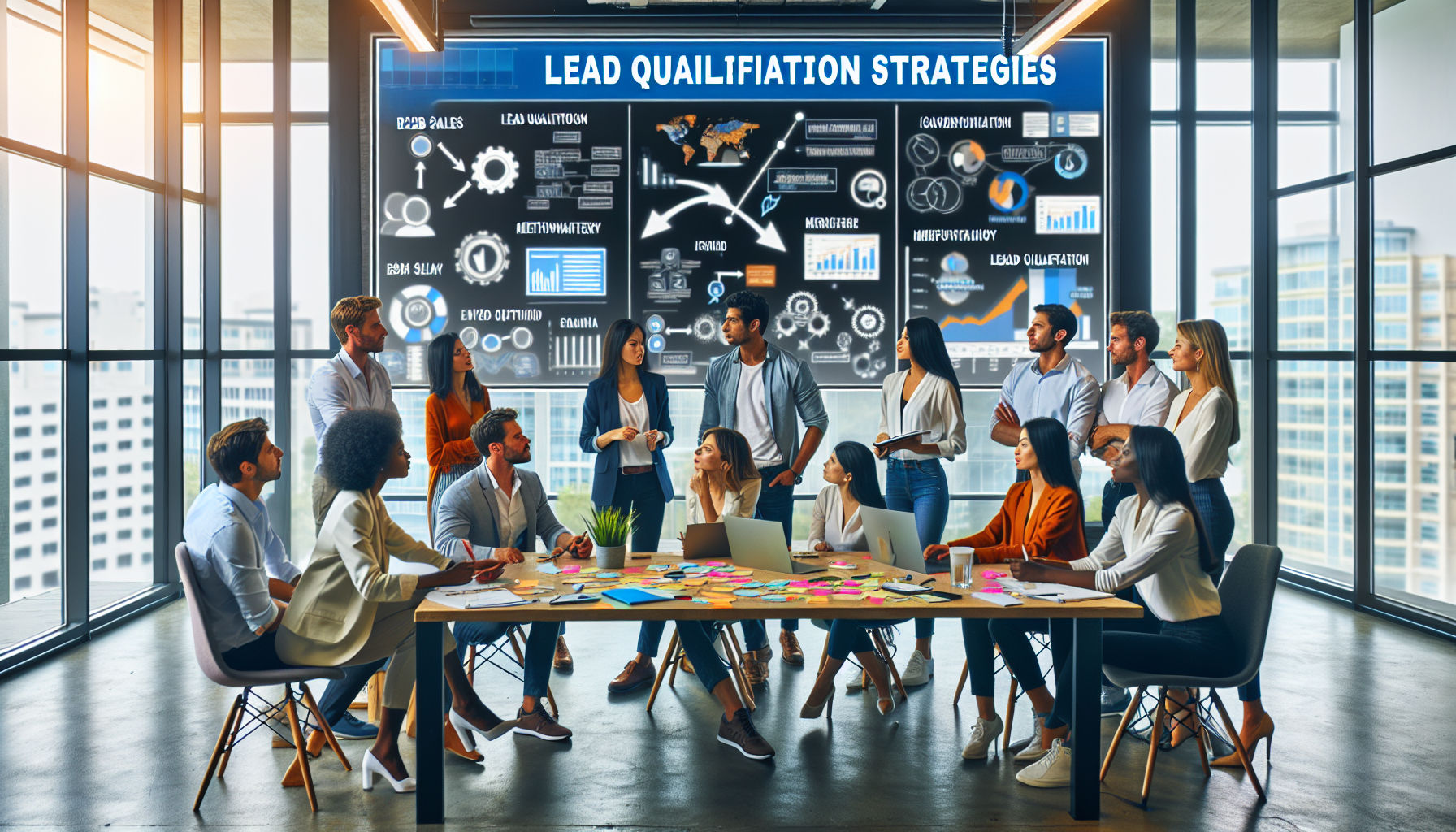
Unraveling the Fundamentals: What Is Lead Scoring?
Lead scoring is a vital tool in B2B sales that helps teams identify which leads might turn into customers. It assigns a score to each lead, showing how likely they are to buy something and how valuable they could be to the business. This system allows sales and marketing teams to focus on the most promising leads, making their work more effective.
Here's why lead scoring is so important:
- Improves Sales Efficiency: By focusing on high-quality leads, companies can use their resources more wisely, which means less time wasted on leads that likely won't result in sales.
- Boosts Conversion Rates: Knowing which leads are most likely to buy helps businesses tailor their approach, leading to more sales. Some businesses see up to a 50% increase in sales because of good lead scoring.
- Better Teamwork Between Sales and Marketing: When both teams use the same scoring system, they work better together. They know which leads to prioritize, which helps align their strategies.
Understanding the basics of lead scoring involves a mix of different types of data:
- Fit Score: This tells if a potential customer fits well with the product or service. It looks at details like the industry they work in, the size of their company, and their job role.
- Intent Score: This score tries to figure out how ready someone is to buy based on how they interact with the company, like visiting the website or downloading content.
These scores come from two main kinds of data:
- Explicit Data: This includes clear, straightforward information like job titles or company size, usually gathered when leads fill out forms or through other direct methods.
- Implicit Data: This data comes from analyzing how leads interact with your website and what they do there, which gives clues about their interest in your product.
Using these insights, businesses can create a lead scoring model that helps them decide which leads to focus on. They start by defining what makes a lead valuable and then assign points based on those criteria. They also set thresholds to determine when a lead is ready to go to the sales team, ensuring only the most promising leads are followed up on.
In summary, lead scoring is all about making the sales process more targeted and efficient. It's not just about finding any customer; it's about finding the right customer. By scoring leads, companies can more easily convert interest into sales, driving revenue and growth.
Understanding Lead Scoring Metrics: Fit and Intent Scores Explained
Lead scoring in B2B sales hinges significantly on two primary components: fit scores and intent scores. These metrics are essential in determining the potential success of converting leads into paying customers. Let's break down why these scores are crucial and how they can significantly enhance sales strategies.
What are Fit Scores?
Fit scores are foundational in identifying whether a potential customer matches well with your product or service. This scoring relies heavily on a variety of data points that categorize a lead's potential based on their company or personal background.
- Firmographic Data: Details such as industry type, company size, and annual revenue are considered to determine if a company fits the ideal customer profile. For example, a tech startup with rapid growth might score highly if your product is geared towards innovative tech companies.
- Demographic Data: Personal attributes like job title and department also play a role. A decision-maker in a relevant field would score higher, indicating a stronger potential for a sale.
What are Intent Scores?
Intent scores measure a lead's likelihood to make a purchase soon, based on their interactions and behaviors related to your business. These are critical in gauging how engaged and interested a potential customer really is.
- Search Data: Keywords that potential customers use can reveal their buying intent. Searching for "pricing plans" might indicate readiness to purchase.
- Website Activity: Frequent visits to certain pages like product demos or pricing information suggest serious buying interest.
- Engagement with Content: Downloading case studies or attending detailed webinars also signifies a high level of interest.
Combining Fit and Intent Scores
Integrating fit and intent scores, known as 2D lead scoring, provides a more comprehensive view of each lead. This method ensures that sales teams do not just target leads that fit the product well, but are also ready to make a purchase decision.
- Calibration of Scores: It's crucial to balance the weight of fit and intent scores to match your specific sales priorities. This might mean adjusting the importance of certain behavioral indicators or demographic details.
Implementing Fit and Intent Scores
To effectively implement these scoring mechanisms, businesses should:
- Define Clear Criteria: Clearly identify what makes an ideal customer and what behaviors strongly indicate buying intent. This helps in assigning accurate scores.
- Use Automation: Employ marketing automation tools to systematically score leads based on the established criteria.
- Regularly Update Scoring Models: Continuously refine and adjust scoring models to respond to new data and changing market conditions to maintain scoring accuracy.
By understanding and utilizing fit and intent scores, sales teams can more effectively prioritize their efforts, leading to improved conversion rates and more efficient resource allocation. This makes the sales process not only faster but also more aligned with the potential for revenue growth.
Diving Deeper: Two-Dimensional (2D) Lead Scoring
Two-dimensional (2D) lead scoring offers a robust method for evaluating potential customers by focusing on two pivotal aspects: their compatibility with your product (fit) and their readiness to purchase (intent). This sophisticated approach ensures that the sales team prioritizes leads that are not only a good match but are also at the right stage in the buying journey.
Key Elements of 2D Lead Scoring
The effectiveness of a 2D lead scoring system hinges on the precise assessment of fit and intent scores.
- Fit Score: This metric determines if a prospect aligns with your ideal customer profile based on firmographic details like industry, company size, and annual revenue. It ensures that the sales resources are concentrated on leads that are most likely to convert.
- Intent Score: This score assesses the likelihood of a lead moving forward in the sales process, based on their interactions such as website visits, content downloads, and demo requests. It gauges a lead's current interest level and readiness to engage further.
How 2D Lead Scoring Enhances Sales Efficiency
By combining fit and intent, a 2D lead scoring system provides a comprehensive outlook on each lead, allowing for better prioritization and resource allocation.
- Integration of Scores: Seamlessly combining fit and intent scores offers a nuanced view of each prospect, highlighting both their eligibility as a customer and their purchase readiness.
- Weighting of Scores: Depending on your business strategy, you can adjust the emphasis on fit versus intent. For instance, a company focused on rapid expansion may prioritize fit to ensure leads have the potential for long-term value.
- Setting Qualification Thresholds: Establish clear benchmarks for when a lead is considered qualified for sales engagement, optimizing the hand-off from marketing to sales.
Implementing a 2D Lead Scoring System
To effectively deploy a 2D lead scoring system, companies need to undertake several strategic steps:
- Gathering Data: Collect extensive quantitative and qualitative data about leads, including both demographic information and behavioral insights.
- Defining Scoring Metrics: Clearly identify what metrics will define fit and intent. This may include specific behaviors, such as engagement with key content or interaction patterns on the website.
- Adjusting Weights: Continually adjust the weights assigned to fit and intent based on evolving market conditions and internal sales feedback.
This structured and strategic approach to 2D lead scoring not only refines the sales process but significantly enhances the potential for closing deals by focusing on the most promising leads first.
Account-Based Marketing (ABM) and Lead Scoring: A Strategic Alignment
Integrating Lead Scoring with Account-Based Marketing
Account-Based Marketing (ABM) shifts the focus from broad marketing initiatives to prioritizing and tailoring marketing efforts directly towards specific high-value accounts. By incorporating lead scoring into ABM, businesses can strategically identify and engage with potential customers that have the greatest likelihood of converting, and whose business goals closely align with the services or products offered.
Qualification at the Account Level
Qualification at the account level is a critical step in the ABM process. It involves evaluating entire accounts — not just individual leads — to determine their potential value to the business. This process uses both demographic and firmographic data to assess whether an account fits the ideal customer profile (ICP). This approach ensures that marketing efforts are concentrated on the accounts that are most likely to drive significant business results.
The Role of Lead Scoring in ABM
Lead scoring in ABM isn't just about ranking potential individual leads; it's about synthesizing information across all relevant contacts within a target organization. This might include scoring based on:
- Behavioral Attributes: Analyses interactions such as content downloads, page visits, and webinar attendance that indicate a lead's engagement and interest level.
- Intent Data: Utilizes both first-party and third-party data to gauge the buying signals and intentions of an account, enhancing the understanding of where they are in the customer journey.
- Demographic and Firmographic Data: Considers essential attributes such as company size, industry, and location, which help in verifying if the account aligns with the ICP.
This multifaceted approach allows businesses to prioritize and dedicate resources to nurturing the accounts with the highest propensity for conversion.
Strategic Benefits of Lead Scoring in ABM
Implementing lead scoring within an ABM framework brings several strategic advantages:
- Enhanced Targeting Efficiency: By focusing on accounts that score highly on both demographic and behavioral scales, businesses can allocate resources more efficiently, reducing time and effort spent on less promising leads.
- Improved Conversion Rates: Accurately scored and prioritized leads are more likely to convert, thus potentially increasing overall sales performance and ROI from marketing campaigns.
- Better Alignment Between Sales and Marketing: Lead scoring sets a uniform criterion for sales and marketing to identify and pursue high-value accounts, fostering collaboration and unified goals across departments.
Implementing Lead Scoring in ABM
To effectively incorporate lead scoring into an ABM strategy, businesses should:
- Define Clear Scoring Criteria: Establish what behaviors, firmographic details, and demographic information correlate strongest with account success. This includes creating customized scoring models for different types of accounts based on their unique traits and needs.
- Leverage Automation and Technology: Utilize advanced CRM and marketing automation tools that can handle complex data inputs and automate scoring processes, ensuring that teams can quickly and efficiently respond to qualified leads.
- Continuous Evaluation and Adjustment: Regularly reassess and fine-tune the scoring model to adapt to changing market conditions or shifts in strategic business objectives.
This structured approach to integrating lead scoring with ABM helps businesses optimize their marketing strategies, leading to more focused efforts and improved outcomes in targeting and converting top-tier accounts.
Exploring Modern Approaches: Product-Led Qualification
In the world of B2B lead scoring, the trend is shifting towards a more interactive approach where the product itself plays a central role in qualifying leads. This method, called product-led qualification, leans on direct user interactions with the product to ascertain a lead's potential. Let's dive into what Product Qualified Leads (PQLs) are, why they matter, and how to effectively score them using this innovative model.
Understanding Product Qualified Leads (PQLs)
A Product Qualified Lead (PQL) is distinguished from traditional leads by their interaction with your product. These leads have not just shown interest but have used your product in ways that signal potential buying intent. Unlike leads judged mainly on demographic or firmographic data, PQLs provide a clearer insight into how leads engage with your product’s value, making them highly valuable for conversion strategies.
Advantages of the PQL Model
The PQL model is favored for several impactful reasons:
- Higher Conversion Rates: PQLs typically show higher conversion rates as they have already recognized the value of the product through direct interaction, unlike those who might still be in the awareness or consideration stage.
- Reduced Friction: By focusing on users already engaging with the product, the PQL model streamlines the sales funnel and reduces the friction often associated with converting top-of-funnel leads who may require more nurturing.
Creating a Lead Scoring Model for PQLs
To tailor a lead scoring model for effective product-led qualification, follow these strategic steps:
- Set PQL Criteria: Define specific actions within the product that signify buying intent, such as frequent logins, usage of key features, or multiple visits to the pricing page. These behaviors suggest that the lead has a high potential for conversion.
- Monitor Product Engagement: Track how leads interact with your product, focusing on metrics like feature adoption, onboarding progression, and activity levels. This data is crucial in determining the lead’s interest and readiness to purchase.
- Dynamically Score Leads: Assign scores based on pre-defined product interaction thresholds. Use automation tools to help scale this process, especially when dealing with a large number of leads.
- Analyze and Adjust: Continuously analyze the engagement patterns of converted leads to refine and calibrate your scoring model, ensuring it adapts to new behaviors and market conditions.
Key Steps to Implement Product-Led Qualification
Implementing a product-led qualification process involves several critical actions:
- Integration with CRM: Ensure that your Customer Relationship Management (CRM) system can track and score lead interactions with your product. Automation is key in handling large volumes of data and interactions efficiently.
- Regular Review and Calibration: Regularly review the scoring criteria and thresholds to ensure they remain relevant and effective in identifying high-quality leads.
- Feedback Loops: Create feedback loops between sales and product teams to continuously improve the product features and the qualification criteria based on lead data and sales outcomes.
By adopting a product-led approach to lead qualification, businesses can focus on leads that have demonstrated a clear interest and engagement with the product, streamlining the sales process and enhancing conversion rates. This method not only optimizes resources but also aligns sales strategies with user behaviors, offering a modern twist to traditional lead scoring systems.
Adapting Lead Scoring for Complex Business Models
For businesses with complex product lines or diverse customer bases, a single lead scoring model may not be sufficient. In such scenarios, multiple lead scoring models are essential to accurately prioritize leads and optimize marketing and sales efforts effectively.
Why Use Multiple Lead Scoring Models?
Complex businesses often require tailored approaches to lead scoring for several reasons:
- Diverse Products and Services: Companies with multiple product lines need separate scoring models to address the unique challenges and opportunities each product presents.
- Varying Customer Segments: Different customer types may have distinct needs and behaviors, necessitating customized scoring strategies to effectively engage each segment.
- Efficient Resource Allocation: By scoring leads based on specific product lines or customer segments, companies can better allocate their resources, ensuring that the right teams are focused on the most promising leads.
Implementing Multiple Lead Scoring Models
Introducing multiple lead scoring models in a business involves several strategic steps:
- Identify Key Customer Segments and Products: Determine the different segments or products that will have their own scoring models.
- Set Specific Scoring Criteria: For each model, define the criteria that will dictate the scoring. This can include demographic information, behavioral data, and engagement metrics.
- Assign Point Values: Points should be assigned to each scoring criterion based on its importance to the customer journey and potential to convert.
- Utilize Automation Tools: Implement marketing automation technologies to facilitate the scoring process, reducing manual effort and increasing accuracy.
- Establish Thresholds for Lead Qualification: Set clear benchmarks that determine when a lead is considered qualified and ready for engagement from the sales team.
- Regularly Review and Optimize: Continuously monitor and adjust the scoring models to ensure they remain accurate and reflect any changes in market conditions or business strategy.
Best Practices for Multiple Lead Scoring
When managing multiple lead scoring models, there are best practices to consider that help maximize their effectiveness:
- Customization: Tailor each model to the specific characteristics and needs of the segment or product it's designed for.
- Negative Scoring: Incorporate negative scores for actions that indicate a lead is less likely to convert, such as unsubscribing from communication.
- Score Decay: Implement a system where scores degrade over time for inactive leads to prioritize engagement with active prospects.
- Alignment with Sales and Marketing: Ensure that the scoring models align with overall sales and marketing strategies to promote collaboration and focus on high-value targets.
- Feedback Loops: Utilize feedback from sales and marketing teams to refine scoring criteria and keep the models aligned with real-world outcomes.
By employing multiple lead scoring models, complex businesses can better handle the intricacies of their operations, ensuring more personalized engagement with leads and ultimately, driving higher conversion rates.
Overcoming Challenges: Pitfalls in Lead Scoring Implementation
Implementing an effective lead scoring system in a B2B environment is crucial for optimizing sales efforts and prioritizing leads efficiently. However, it's not without its challenges and pitfalls. Here we'll explore some common issues that businesses face when implementing lead scoring and offer practical solutions to overcome these hurdles.
Neglecting Data Quality
One major challenge is the reliance on inaccurate or incomplete data which can skew the assessment of lead quality.
- Solution: Prioritize enhancing data quality by routinely cleaning and updating your database, enforcing data validation rules, and perhaps investing in data enrichment services to fill gaps and boost lead profiles.
Complexity in Scoring Models
Creating overly intricate scoring models can lead to difficulties in implementation and maintenance.
- Solution: Simplify your scoring model, focusing on pivotal factors like engagement level, company size, and industry relevance. Regularly revise and refine your scoring criteria to keep them relevant and manageable.
Ignoring Behavioral Data
Focusing solely on demographic data while neglecting the insights that can be gained from lead behavior is a common oversight.
- Solution: Integrate behavior-based metrics into your model, including website visits, content downloads, and social media interactions to gain a holistic view of a lead's interest and intent.
Lack of Collaboration Between Sales and Marketing
Disconnection between sales and marketing teams can significantly hinder the effectiveness of lead scoring.
- Solution: Foster a collaborative environment by establishing a shared understanding of what qualifies as a lead and maintaining open communication channels for continuous feedback. Regular meetings and reports can help align both teams.
Poor Communication and Misalignment
Ineffective communication can lead to a mismatch in lead generation and scoring strategies, causing further inefficiencies.
- Solution: Ensure effective communication and strategic alignment between sales and marketing teams. Develop a shared system of lead scoring to enhance collaboration and efficiently identify high-intent leads.
Static Scoring Models
Using unchanging scoring models that do not adapt to new data or market shifts can result in missed opportunities.
- Solution: Employ dynamic scoring models that evolve with changing market conditions and feedback from sales and marketing to capture emerging trends and customer preferences.
Overemphasis on Demographics
Relying too heavily on demographic data can be limiting, as it might overlook the nuanced behaviors and preferences of leads.
- Solution: Utilize a mixed approach that includes both demographic and behavioral data, enriching the profiling process and enhancing the predictive power of your scoring system.
Difficulty in Measuring ROI
Another challenge is the ambiguity in measuring the return on investment (ROI) from lead scoring systems, which can make it difficult to justify the effort and resources allocated.
- Solution: Establish clear Key Performance Indicators (KPIs), such as conversion rates and sales revenue growth, to measure the effectiveness of your lead scoring efforts. Regular reviews of these metrics will help refine your approach and demonstrate value.
By addressing these challenges with practical and strategic solutions, businesses can enhance their lead scoring systems, leading to better resource allocation, improved conversion rates, and stronger alignment between sales and marketing teams.
Harnessing Technology: Advanced Lead Scoring Techniques
Today, advanced lead scoring techniques are revolutionizing how businesses prioritize and interact with potential customers. By leveraging the power of predictive scoring, which incorporates machine learning and artificial intelligence, companies can improve both the accuracy and efficiency of their lead scoring processes. Let's delve into how these innovative technologies are shaping the future of lead scoring.
Predictive Scoring with Machine Learning and AI
Predictive lead scoring is an advanced approach that uses algorithms to predict the likelihood of a lead converting into a customer. Unlike traditional methods, this approach makes use of extensive data analysis and is capable of identifying patterns that are not obvious in manual systems.
- Data Collection: It utilizes a broad range of data points including demographic information, behavioral data like website interactions and email engagement, and firmographic details such as company size and industry.
- Machine Learning Algorithms: These sophisticated algorithms, such as decision trees and neural networks, analyze historical data to find correlations between various attributes and conversion outcomes.
- Model Training and Validation: The accuracy and reliability of predictive models are ensured through rigorous training on historical data and subsequent validation against test data.
- Continuous Optimization: Predictive models are not static; they continually learn from new data, refining and enhancing scoring accuracy over time.
Benefits of Predictive Scoring
Adopting predictive scoring offers several key advantages:
- Improved Accuracy: These models capture subtle nuances in lead behavior, offering more precise predictions than traditional scoring.
- Automated Scoring: With AI-driven scoring, the process becomes automated, reducing the manual workload and allowing sales teams to concentrate on the most promising leads.
- Enhanced Sales and Marketing Alignment: A data-driven view of lead quality fosters better coordination between sales and marketing teams, ensuring unified efforts in targeting high-quality leads.
Implementing Predictive Scoring
To implement these advanced scoring techniques, businesses should consider the following strategies:
- Collaboration: Work closely with both sales and marketing teams to determine the key attributes that influence conversion, ensuring that the model aligns with overall business objectives.
- Data Quality: High-quality data is critical for training reliable models. Ensure the data used is comprehensive and accurate to avoid biased or incorrect outputs.
- Choosing the Right Tools: Opt for advanced lead scoring tools that support customization and real-time scoring, such as Salespanel, which offers AI-driven predictive scoring capabilities.
- Continuous Monitoring and Refinement: Establish a feedback loop with sales teams to continuously refine the scoring criteria based on real-world performance and outcomes.
By embracing these advanced lead scoring methods, companies can not only streamline their lead management processes but also significantly enhance their conversion rates, driving more effective sales and marketing strategies.
Learning from Success: Case Studies of Effective Lead Scoring
Exploring real-world examples of businesses that have successfully implemented lead scoring provides valuable insights into best practices and the significant impacts of these strategies. Let's delve into several case studies that illustrate the effectiveness of well-executed lead scoring systems.
Case Study: X Education - Online Course Provider
X Education, a provider of online courses for industry professionals, faced challenges in converting leads into paying customers. Recognizing the need for a data-driven approach, they implemented a comprehensive lead scoring model.
- Lead Collection: X Education collected leads through various channels including website forms, referrals, and search engine marketing.
- Lead Scoring Model: They developed a model assigning scores based on the likelihood of conversion, aiming to boost their conversion rate significantly from 30% to around 80%.
- Data Points Considered: The scoring system utilized both explicit data like company size and industry, and implicit data such as website behavior and email interactions.
- Outcome: The implementation of this targeted lead scoring model enabled X Education to prioritize leads more effectively, resulting in a substantial increase in conversion rates.
Case Study: SaaS Product Company
A software as a service (SaaS) company in a highly competitive market capitalized on lead scoring to better identify and prioritize potential customers, enhancing their sales strategy.
- Objectives: The company focused on trial usage, feature exploration, and user engagement with support documentation to understand user behavior.
- Scoring Model: Points were assigned for specific actions such as signing up for a free trial (+20 points), expressing interest in a product demo (+25 points), and using the knowledge base (+15 points).
- Results: By scoring leads based on meaningful interactions, the company streamlined its sales approach, leading to improved communication strategies and increased conversion rates.
Case Study: B2B Company with Diverse Client Base
A B2B company with a variety of clients used lead scoring to enhance their sales efficiency by better identifying high-value leads.
- Key Strategies: Prioritization was based on company size and industry relevance, with points awarded for engaging with whitepapers (+15 points), expressing interest in a demo (+30 points), and participating in webinars (+25 points).
- Impact: This targeted approach allowed the company to focus efforts on high-potential leads, resulting in improved conversion rates and more efficient sales processes.
Common Themes and Best Practices
Across these case studies, several recurring themes and best practices emerge:
- Integrated Client Profiles: Combining multiple data sources to create comprehensive lead profiles helps in delivering personalized experiences and targeted marketing.
- Behavioral Signals: Monitoring actions like page visits and content downloads provides crucial insights into buying intent, vital for scoring in B2B sales.
- Predictive Lead Scoring: Implementing machine learning tools to refine scoring models ensures they remain effective and relevant by adapting to changing data patterns.
- Continuous Monitoring and Adjustment: Regularly evaluating the performance of scoring models and adjusting them based on feedback and sales metrics is essential for maintaining their effectiveness.
These case studies demonstrate that when implemented correctly, lead scoring can drastically improve the efficiency of sales and marketing efforts, leading to higher conversion rates and significant increases in revenue.
Best Practices for Successful Lead Scoring Implementation
Defining and Understanding Your Ideal Customer Profile
One of the keys to successful lead scoring is having a clear understanding of who your ideal customer is. This involves gathering significant demographic and firmographic details that help in creating a comprehensive profile. Important attributes to consider include:
- Job title and role in the company
- Company size and industry
- Technological readiness and tools currently used
These details provide the foundation for setting up effective scoring criteria that align with your business targets.
Setting Up Effective Scoring Criteria
To set up an effective lead scoring system, it’s vital to select and weight various attributes based on their importance:
- High-Value Actions: Assign higher scores to behaviors that indicate strong buyer intent, such as visiting pricing pages or requesting product demos.
- Negative Scoring: It's also crucial to implement negative scoring for behaviors that suggest a lower likelihood of conversion, for instance, ignoring emails.
These criteria help in distinguishing between leads that are ready to convert and those that need more nurturing.
Aligning Sales and Marketing Goals
Effective lead scoring relies heavily on alignment between sales and marketing teams. Collaborative efforts are essential for defining what constitutes a 'qualified lead' and ensuring that both teams work towards the same goals. Regular meetings and strategy sessions can help maintain this alignment.
Establishing Clear Thresholds for Lead Qualification
Defining a clear threshold for lead qualification ensures that only the most promising leads are forwarded to sales. This score threshold should be set based on the scoring criteria previously established and should be indicative of a lead’s readiness to purchase or engage further.
Integrating Lead Scoring with Existing Systems
Your lead scoring system should seamlessly integrate with existing CRM and marketing automation tools. This integration allows for automatic updates and sharing of lead scores across departments, ensuring everyone has access to real-time data.
Choosing the Right Lead Scoring Software
Selecting suitable lead scoring software is critical for automating and managing the scoring process effectively. Look for software that offers:
- Ease of integration with existing CRM systems
- Capability to handle multiple scoring models
- Scalability as your company grows
Continuous Optimization and Refinement
Lead scoring is not a set-and-forget system; it requires ongoing adjustments and optimizations based on performance data and changing market conditions. Utilizing AI and machine learning can help refine the scoring models, ensuring they remain accurate and effective over time.
By diligently implementing these best practices, businesses can set up a lead scoring system that not only identifies the most promising prospects but also enhances the overall efficiency of their sales and marketing efforts.
Wrapping it Up: Maximizing Your Lead Scoring Strategy
Lead scoring is a powerful tool for enhancing the efficiency and effectiveness of your sales and marketing efforts. By understanding and implementing advanced lead scoring strategies, businesses can prioritize their prospects better, streamline their marketing efforts, and ultimately increase conversions.
Throughout this article, we've explored diverse aspects of lead scoring, including the importance of fit and intent data, two-dimensional lead scoring, and the significance of account-level scoring for Account-Based Marketing (ABM). We've also delved into product-led qualification with Product Qualified Leads (PQLs) and the necessity of multiple lead scores for businesses with complex models.
Key Strategies to Enhance Lead Scoring
Integrating various lead scoring approaches can significantly amplify your sales processes. Here are some strategic tips to maximize your lead scoring efforts:
- Integrate Fit and Intent Scores: Combining both fit and intent scores ensures that you target leads who are not only a good fit for your product but are also at the right stage in their buying journey. This multi-dimensional approach can vastly improve lead prioritization and resource allocation.
- Focus on Account-Level Scoring: For B2B companies, especially those in the ABM space, scoring at the account level can be crucial. This approach helps in catering uniquely to high-value accounts, enhancing personalization and engagement.
- Leverage Product Data for PQLs: In product-led growth strategies, use product interaction data to score leads. This can be a more direct indicator of a lead's interest and potential for conversion, especially in tech and SaaS industries.
- Adopt Multiple Scoring Models: If your business offers multiple products or services, or serves diverse markets, consider implementing separate scoring models. This specialization allows for more precise targeting and effective marketing.
- Regularly Update Your Scoring Models: Lead scoring is not a one-time setup. It's vital to continually adjust your scoring models based on new data, market trends, and changes in business strategy.
By leveraging these strategies, businesses can make their lead scoring systems more dynamic and responsive to the needs of both their sales teams and their potential customers.
Conclusion
Effective lead scoring is essential for optimizing marketing efforts and prioritizing leads that are most likely to convert. From using technology like AI and machine learning to adopting innovative scoring models, businesses have numerous tools at their disposal to enhance their lead qualification processes. By focusing on strategic lead scoring implementation and continuous improvement, companies can not only increase their operational efficiency but also boost their sales outcomes significantly.
Related publications
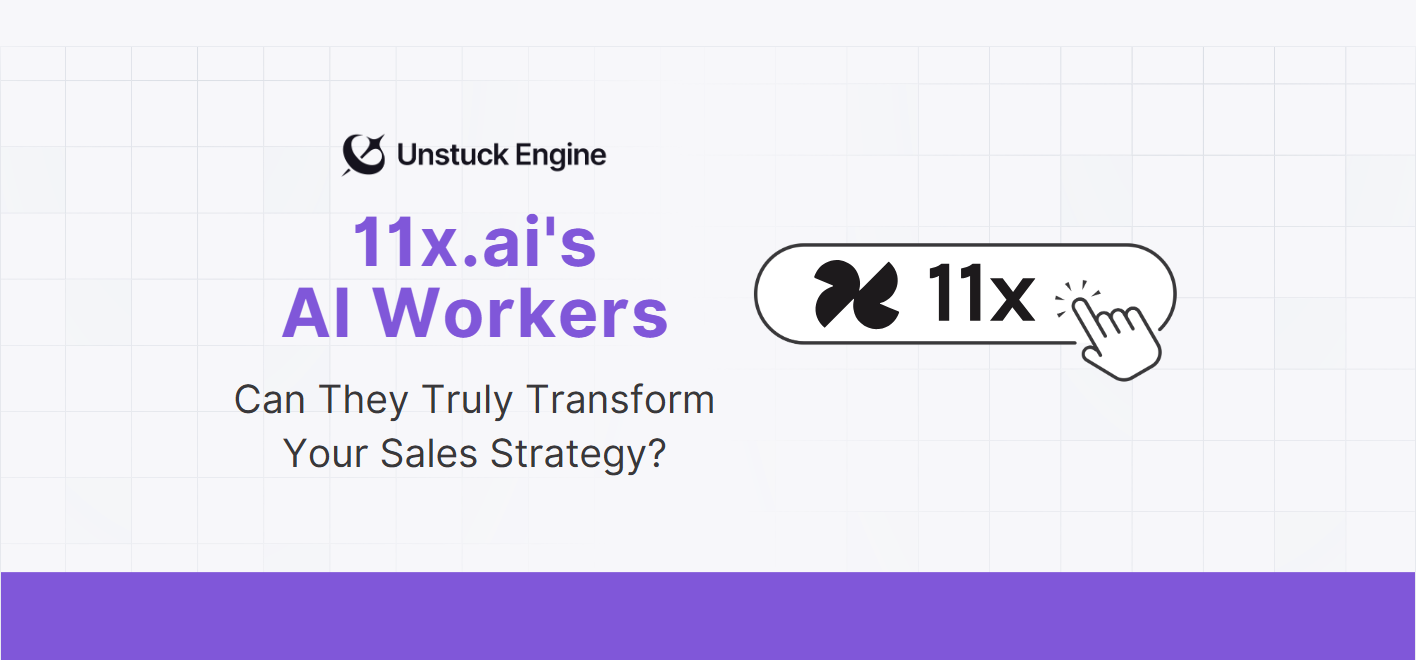
11x.ai's AI Workers: Can They Truly Transform Your Sales Strategy?
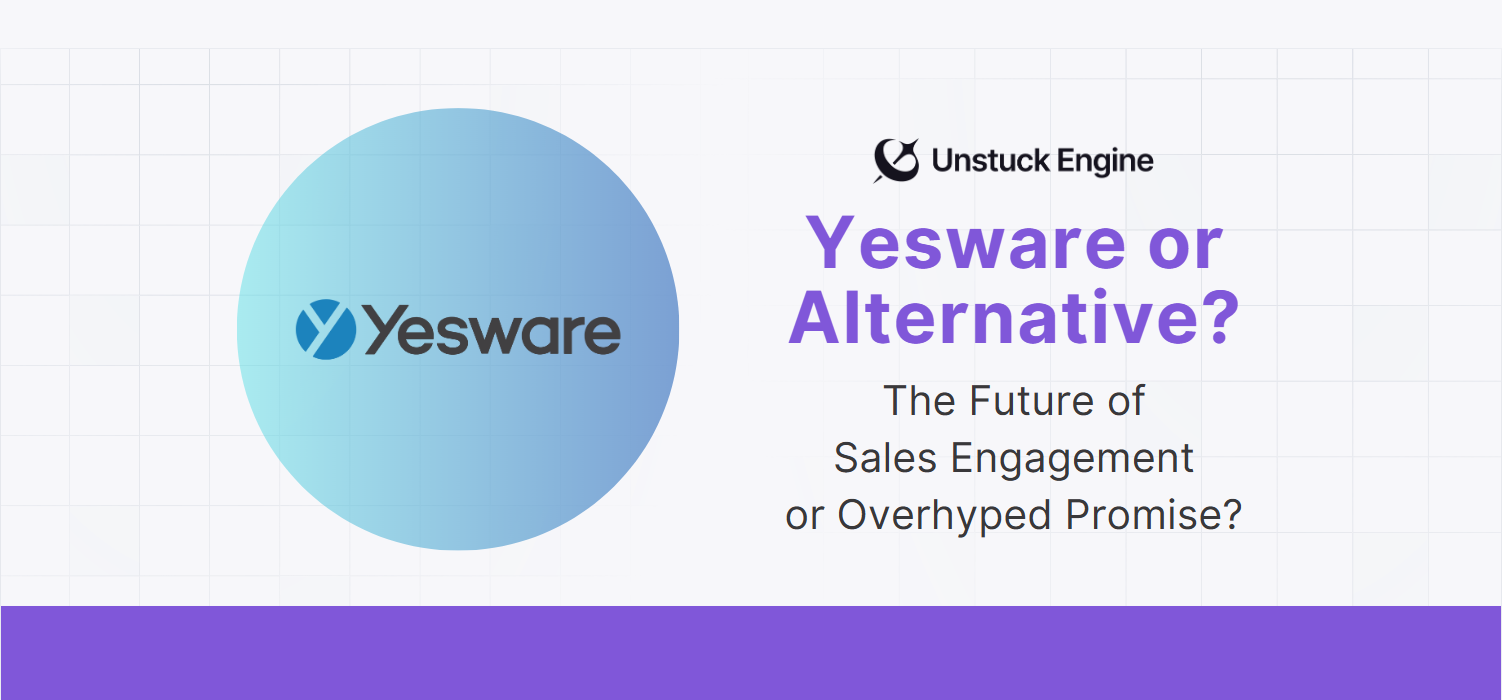